This blog post presents the finding of Communitor's Crowd-Mapping of Community-based Monitoring and Citizen Science initiatives in the Global South. We analyze the objectives, designs, degrees of citizens or communities’ participation, and main data users in these initiatives.
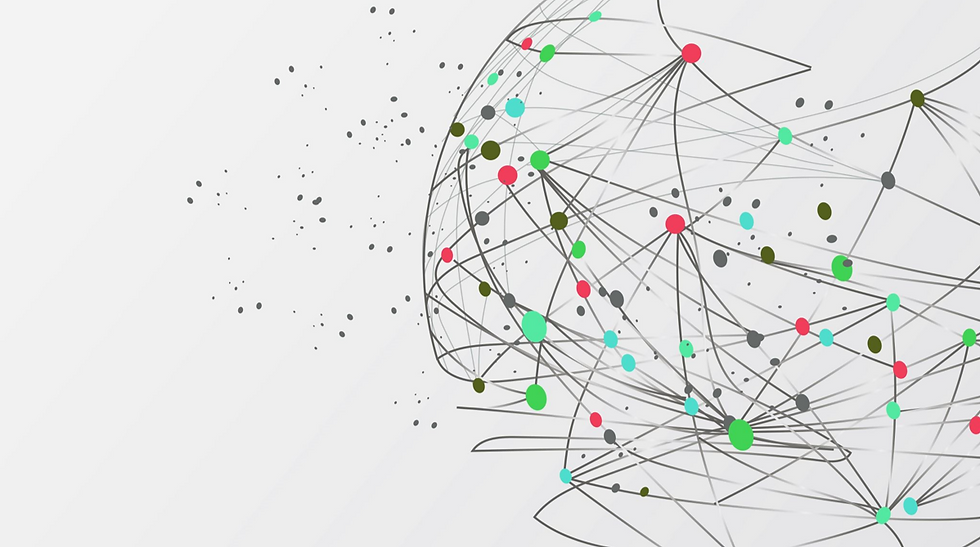
Over the past decades, Community-Based Monitoring (CBM) and Citizen Science (when we these two terms together we abbreviate them to CMCS) have become recognized interventional approaches for better public governance and development. They are continuously used as innovative tools for enhancing citizen engagement in governance and research (Rask et al., 2018). CS refers to the active involvement of citizens in scientific research. Researchers collaborate with citizens to produce knowledge that can be used for scientific purposes as well as community development (Vohland et al., 2021). The same collaborative mechanisms used in CS are used in CBM initiatives. CBM involves a combination of tools employed by users of public services or resources to generate information for learning and assessing interventions' processes and outcomes to improve service delivery, resource management and accountability (Björkman & Svensson, 2009; Waddington et al., 2019; The Global Fund, 2020). In other words, CS and CBM are learning and social accountability mechanisms or tools.
Despite the similarities, there is a disconnection between the literature on CBM and CS. This disconnection obstructs learning from the findings of research on both initiatives. Moreover, the CMCS literature often focuses on theoretical aspects or case studies. There are several systematic reviews and meta-analyses (e.g. Andrachuk et al., 2019; Klonner et al., 2016; Kouril et al., 2016; Molina et al., 2016; Waddington et al., 2019); however, an overview, across countries and fields, of what is happening on the ground is lacking. We attempt to fill this literature gap by identifying, together with the other members of our Communitor, CMCS projects in the Global South and mapping their geographic locations, fields, and intervention designs. This blog explains our identification and analysis methodology and the main findings. We presented this research results in one of the Member of the Month and the recording can be found here. Moreover, the interactive slides of this presentation provides a good visualization of the analyzed data and this blog post makes reference to them.
Purpose and Methodology
We decided to conduct this mapping exercise with our community of practice during the Communitor’s launching event. The aim of this exercise was straightforward: to use the power of our community to map the CMCS initiatives in the Global South countries. Communitor brings together members whose activities or interests are related to CMCS; therefore, it is a perfect network for conducting such an exercise. We created an excel file with two sheets on google drive. The first sheet was the questionnaire for our participants. We allowed anyone with the link to edit the file but asked the participants to avoid editing or removing someone else’s entries. The second sheet was a metadata file for quick guidance about the questions to respond to, concepts, and classification levels. Initially, our questionnaire file included a wide range of questions. However, we decided to reduce it since it would have required too much expertise and time for our participants to fill in the information. The final questions were about the initiatives’ title, country, implementation period, and field. We also asked the participants to describe the interventions and indicate any website links to online resources. Finally, the participants had to assess the importance of accountability and learning as objectives and identify the interventions’ designs and data users using the levels listed in the drop-down menus. We have written a blog post on our website explaining all details and instructions about this exercise and shared it through our social media channels.
Contributors, collected data, and analysis
In total, 7 participants identified 47 CMCS initiatives. Only 2 participants (Dr. Selmira Flores and an IOB student Queenie Diane Malabanan) were not members of the Outreach Team (Doreen Kyando, Diana Tiholaz, Narayan Gyawali, Odaro Jude, and Solomon Mwije). The authors of this analysis included the highest number of initiatives (Solomon Mwije=19 initiatives and Diana Tiholaz=12 initiatives). Apart from Solomon Mwije, Diana Tiholaz, and Queenie Diane Malabanan, the members described the projects they are or were involved in. The other three participants included projects they know from scientific articles, reports, and grey literature. Hence, this is not a systematic mapping, and we have to consider this fact when we interpret the results of this analysis. We analyzed the collected data in Stata and used Flourish to visualize it.
Results
CBCS initiatives on the World Map
Our 47 projects are from 18 countries from three continents (Asia, Africa, and Latin America) (see slide number 6). 6 of these projects were multi-countries (see slide number 8). Sub-Saharan Africa is the most represented region (41 projects). Uganda is the most represented country with 25 initiatives, followed by Tanzania with 11 initiatives. This fact is easily explained by the main contributors’ origins and geographic interests. Therefore, our data and the way we collected it do not allow us to say that Uganda is a country where CMCS projects are often implemented. However, one of the authors conducted recently a literature review of community-based monitoring impact evaluations and found Uganda a popular country to implement such projects (30% of the analyzed studies).
Fields of intervention
To facilitate the analysis of interventions' fields, we used Musgrave’s classification that divides the goods into 4 categories based on two criteria: exclusivity and consumption (Desmarais-Tremblay, 2014). Following this classification, a public good is non-excludable (exclusion of other consumers is not feasible) and non-rival consumption (the joining the consumption by additional citizens does not make it more difficult for the existing consumers to benefit from it). A simple example is street lighting. On the opposite side, we have the private goods excludable and rival. Between these two types of goods, there are the club goods (e. g. the benefits of being a cooperative member) and common pool resources (e. g. fish stock). Besides, we distinguished between public goods (when citizens benefit from them) and public bad (when citizens suffer because of them, e.g. diseases). Even if this is a straightforward classification, we had some difficulties differentiating between public goods and common pool resources. For instance, the delivery of water services is a public good as long as there are enough water resources. As soon as the water resources start to be depleted (e.g. because of population growth or climate change), every additional consumer crowds out the use of this service, and the public goods transform into a common pool resource.
As expected, most of the identified CMCS initiatives aimed at monitoring public goods (32 initatives) (see slide 8). 8 initiatives monitored common-pool resources, and 7 initiatives for public bads. We further divided these categories into public services, environment, poverty and development, agriculture, and natural disasters. Considering these sub-categories, most of the initiatives (25) fall in the public services sub-category, followed by the environment ones (15 initiatives). We do not find this aspect surprising since all contributors are researchers or practitioners in the field of governance and public service delivery.
Interventions’ start and duration
We did not observe any pattern linked to the intervention field and start (see slides number 10-13). Though, there are two picks for the start of these interventions, the beginning and end of the 2010s decade. Keeping in mind our data's bias, this observation might be related to the popularity of transparency and accountability initiatives at the end of the 2000s (McGee &Gaventa, 2010) and the citizen science concept starting with the middle of the previous decade. On average, a CMCS intervention lasts 3.77 years, but the mode is 1 year (13 interventions) (see slide 14). This means that most of the interventions are very short. We arrive to the same conclusion when we differentiate between ended and ongoing initiatives (see slide 15). These short interventions seem to be pilot or short research projects. Hence we cannot expect them to produce major and long lasting changes. The most long-lasting initiative in our dataset is Community based monitoring evaluation system (CBMES) program in Uganda implemented by the Uganda Debt Network that started in 2001 and is still ongoing.
Objectives: Learning vs Accountability
Like evaluation, the CMCS follow two main objectives: learning and accountability. Learning refers specifically to the embedment of local or indigenous knowledge into policy-making or research. Accountability is more specific to CBM and refers to strengthening politicians, civil servants, and public service providers to be responsible for their actions and respond to citizens' needs. The relationship between these two objectives in the literature is vastly contested. Reinertsen et al. (2022) identify four positions in this debate, namely, these two objectives are: (1) complementary; (2) in reconcilable tension; (3) opposing (problematic trade-off situation); and (4) irreconcilable.
Our participants were asked to appreciate learning importance on a 5 levels scale (from 1 - Not at all important to 5 - Extremely important). Learning was appreciated either 4 - Very important or 5 -Extremely important for most of the initiatives (see slide 18). The learning level mean is 4,49. Only one initiative aiming to monitor the forests in Ethiopia to prevent deforestation, received the score 2 - Slightly important. We cannot say the same about accountability. Only 7 interventions received the maximum score for accountability importance and the mean score is 2.64 (see slide 19). When it comes to learning accountability relation, we observe a trade-off, but the correlation coefficient is relatively low, (-0,271) (see slide 20).
Relations with the state
Most of the CMCS initiative’s designs entail establishing either collaborative or confrontational relations with the state. Collaborative design means that the intervention attempts to engage citizens and government representatives jointly in monitoring the service delivery or natural resources. Confrontational design strongly encourages citizens to hold government official and public service providers accountable through different activities (e. g. dedicated accountability meetings). 33 interventions in our dataset developed a collaborative relationship with the state actors, 4 - a confrontational one, and 10 - no relation at all (see slide 21). The initiatives with no relation with the state were academic research projects focused on data collection. Our dataset also suggests that initiatives with confrontational designs have accountability as an important objective; while the ones with no relations with the state did not focused on accountability strengthening (see slide 22).
Presence of a dedicated monitoring team
There is a wide range of obstacles for citizens participation, such as information gap, collective action, opportunity costs, low perceived efficacy (see Molina et al., 2016; Waddington et al., 2019; Dewachter & Holvoet, 2017). Presence of a monitoring group aims to deal with part of these obstacles. The downside of this solution is the risk of transforming the monitoring group toa gatekeeper that obstructs inclusion. 37 of our identified interventions previewed a monitoring group and 10 did not (see slide 24). We did not observe any trend related to period when it comes to a monitoring group presence. But we notice that all CMCS initiatives in the field of common pool resources include a monitoring group (see slide 25).
Community or citizens participation
CMCS preview different community or citizens' degree of participation. To map our initiatives we distinguished between 4 levels: (1) Crowd monitoring - externally driven with local data collectors; (2) Distributive intelligence - collaborative monitoring with external data interpretation; (3) Participatory science - collaborative monitoring with local data interpretation; (4) Extreme citizen science - researchers and citizens are involved together in all projects' stages; and (5) Autonomous local monitoring (Danielsen et al, 2009; Haklay, 2013). Around 30% of our initiatives are distributive intelligence and 30% participatory science (see slide 26). 21% belong to crowd monitoring initiatives category while 10% and 6% are extreme citizen science and autonomous local monitoring, respectively. We observe a positive correlation between the importance of accountability and degree of participation, the correlation coefficient is 0,5873 (see slide 27). This finding makes sense, it is easy to assume that accountability strengthening require a high degree of citizens’ participation. However, we do not notice any relation between the degree of community participation and importance of learning, intervention duration and interaction with the state (see slide 28-30).
Data users
We mapped the users of the data produced by these initiatives. Most initiatives had multiple data users, as expected (see slide 31-33). The state and the local community are the most often users and this is a positive finding, considering the importance of learning as an objective. If the academia and civil society had been the main data users, then the risk of information extraction would have been high. Only four initiatives had academia as the only data user (see slide 32).
Conclusion
We conducted this mapping exercise to elicit an overview of CMCS initiatives across countries and fields. Most of the CMCS interventions identified by our mapping exercise are collecting data on public goods and, more precisely, public services. The majority of them are also very short, from 1 to 3 years, signifying that these are pilot interventions or short research projects and hence have a diminished capacity to lead to long-term changes within the communities and society. In terms of objectives, learning was rated very or extremely important for most initiatives, while accountability received a rather average assessment on our importance scale. We observe a trade-off between these two objectives; however, the correlation coefficient is relatively low. A collaborative relationship with the state is the most favored one. Our dataset also suggests that initiatives with confrontational designs aim at accountability strengthening, while the ones with no relations with the state did not see accountability as an objective. Most interventions include a monitoring group that collaborates with the researchers to collect data, and the community is at least partially involved in other project activities. Finally, most interventions allow multiple users to access the generated data and findings.
In conclusion, our analysis suggests that CMCS are viewed as learning tools for communities and state actors, mainly in public service delivery. The popularity of this approach seems to be influenced by international institutions’ initiatives and trends in the research agenda rather than local initiatives. Besides CMCS has not yet become a solid traditional approach to engaging communities in governance and research. Many of the promises of these interventions have little chance to be achieved due to their short implementation period and relatively limited participation of citizens and communities in other intervention stages than data collection. Taking into account the limitations of our data, it is difficulty, however, to confirm the findings of this exercise. To obtain a comprehensive overview of CMCS in practice, we recommend conducting a systematic scoping and review involving larger teams of researchers and using methodological designs that provide primary data.
References
Andrachuk, M., Marschke, M., Hings, C., & Armitage, D. (2019). Smartphone technologies supporting community-based environmental monitoring and implementation: a systematic scoping review [Review]. Biological Conservation, 237, 430-442. https://doi.org/10.1016/j.biocon.2019.07.026
Björkman, M., & Svensson, J. (2009). Power to the people: Evidence from a randomized field experiment of a community-based monitoring project in Uganda. Quarterly Journal of Economics, 124(2), 735–769.
Danielsen, F., Burgess, N.D., Balmford, A., Donald, P.F., funder, m., jones, j.p.g., alviola, p., balete, d.s., blomley, t., brashares, j., Child, b., enghoff, m., fjeldså, j., holt, s., hübertz, h., jensen, a.e., jensen, p.m., Massao, J., Mendoza, M.M., Ngaga, Y., Poulsen, m.k., rueda, r., sam, m., skielboe, t., stuart-hill, g., Topp-Jørgensen, E. & Yonten, D. (2009), Local Participation in Natural Resource Monitoring: a Characterization of Approaches. Conservation Biology, 23, 31-42. https://doi.org/10.1111/j.1523-1739.2008.01063.x
Desmarais-Tremblay, M. (2014). On the Definition of Public Goods. Assessing Richard A. Musgrave’s contribution. In Centre d’Economie de la Sorbonne, Working paper: HAL Id: halshs-00951577.
Dewachter, S., & Holvoet, N. (2017). Intersecting social-capital and perceived-efficacy perspectives to explain underperformance in community-based monitoring. Evaluation, 23(3), 339-357. https://doi.org/10.1177/1356389017716740
Haklay, M. (2013). Citizen Science and Volunteered Geographic Information: Overview and Typology of Participation. In D. Sui, S. Elwood, & M. Goodchild (Eds.), Crowdsourcing Geographic Knowledge: Volunteered Geographic Information (VGI) in Theory and Practice. (pp. 105–22) Springer. https://doi.org/10.1007/978-94-007-4587-2_7
Klonner, C., Marx, S., Uson, T., de Albuquerque, J. P., & Hofle, B. (2016). Volunteered Geographic Information in Natural Hazard Analysis: A Systematic Literature Review of Current Approaches with a Focus on Preparedness and Mitigation [Review]. Isprs International Journal of Geo-Information, 5(7), 20, Article 103. https://doi.org/10.3390/ijgi5070103
Kouril, D., Furgal, C., & Whillans, T. (2016). Trends and key elements in community-based monitoring: a systematic review of the literature with an emphasis on Arctic and Subarctic regions. Environmental Reviews, 24(2), 151-163. https://www.jstor.org/stable/envirevi.24.2.151
McGee, R. & Gaventa, J. (2010). Review of Impact and Effectiveness of Accountability and Transparency Initiatives: Synthesis Report. Department for International Development.
Molina, E., Carella, L., Pacheco, A., Cruces, G., & Gasparini, L. (2016). Community monitoring interventions to curb corruption and increase access and quality of service delivery in low- and middle-income countries: a systematic review. Campbell Systematic Reviews, 8. https://doi.org/10.4073/ csr.2016.8
Reinertsen, H., Bjørkdahl, K., & McNeill, D. (2022). Accountability versus learning in aid evaluation: A practice-oriented exploration of persistent dilemmas. Evaluation, 28(3), 356–378. https://doi.org/10.1177/13563890221100848
Rask, M., Mačiukaitė-Žvinienė, S., Tauginienė, L., Dikčius, V., Matschoss, K., Aarrevaara, T., & D'Andrea, L. (2019). Public Participation, Science and Society: Tools for Dynamic and Responsible Governance of Research and Innovation. Routledge.
The Global Fund. (2020). Community-based monitoring: An Overview. Geneva: The Global Fund.
Vohland, K., Land-zandstra, A., Ceccaroni, L., Lemmens, R., Perelló, J., Ponti, M., Samson, R., & Wagenknecht, K. (2021). The science of citizen science. 1st ed. Springer International Publishing.
Waddington, H., Sonnenfeld, A., Finetti, J., Gaarder, M., John, D., & Stevenson, J. (2019). Citizen engagement in public services in low- and middle-income countries: A mixed-methods systematic review of participation, inclusion, transparency and accountability (PITA) initiatives. Campbell Systematic Reviews, 15(1-2), e1025. https://doi.org/https://doi.org/10.1002/cl2.1025
Comments